Artificial intelligence and emerging regulatory expectations
Artificial intelligence and emerging regulatory expectations
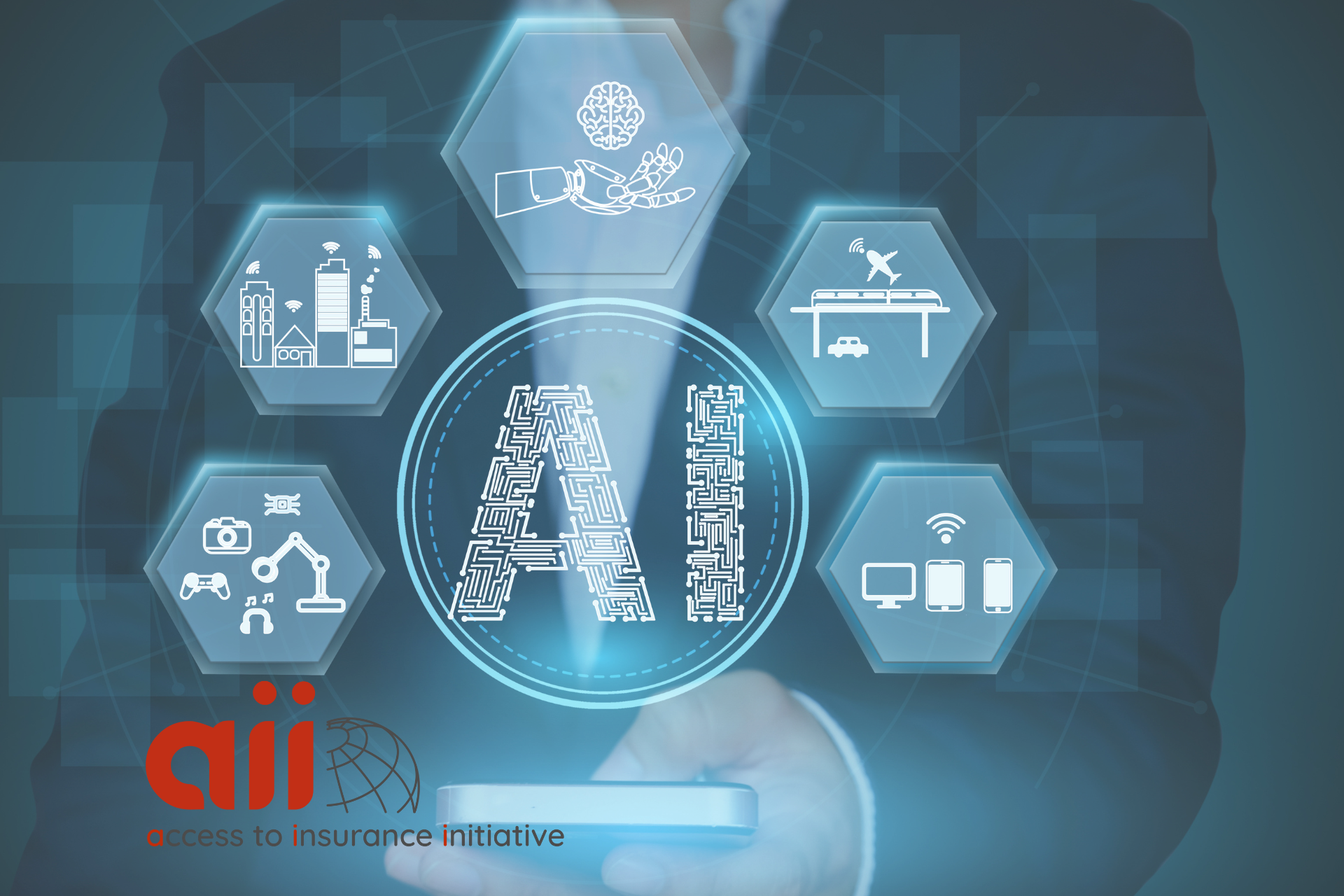
Artificial Intelligence (AI), including Machine Learning (ML), is one of the technologies reshaping the financial sector, including insurance. AI has the potential to significantly improve the delivery of financial services to consumers as well as the operational and risk management processes within firms, which can present many opportunities for expanding financial inclusion. On the flip side, the complexities of AI could overshadow these benefits, as it may exacerbate and introduce new risk exposures, especially around transparency and fairness. For these reasons, clear guidelines and tailored regulatory responses are necessary to mitigate the potential negative consequences of AI-driven solutions to protect consumers and firms.
Supervisors are increasingly working towards developing mechanisms that will protect both the consumer and the firms against AI's possible challenges while using its potential. The European Commission published in April 2021 the first proposal on regulations regarding this issue. Several other national authorities have also issued guidelines, principles and discussion papers on the topic. Some examples include the 2020 European Banking Authority Report on big data and advances analytics, and the 2021 Federal Financial Supervisory Authority of Germany’s report on big data and artificial intelligence, among others (see page 8 for examples).
On 7 April 2022, the A2ii and the IAIS hosted a supervisory dialogue based on the Financial Stability Institute (FSI) paper Humans keeping AI in check – emerging regulatory expectations in the financial sector by Jeffery Yong and Jermy Prenio, published in August 2021.
During the dialogue, Jeffery Yong (FSI) presented the insights from this paper. Julian Arevalo from the European Insurance and Occupational Pensions Authority (EIOPA) presented EIOPA's report on Governance Principles for AI. In the afternoon session, they were joined by Awelani Rahulani from the South Africa Financial Sector Conduct Authority (FSCA), who presented a case study on Data EQ - a tool that analyses online customer conversations to optimise social customer service, generate new CX (customer experience) insights, manage risk and improve regulatory reporting.
FSI: Humans keeping AI in check – emerging regulatory expectations in the financial sector
As argued in FSI's paper, the common principles evolving around AI regulation can be summarised in 5 themes or areas:
1. Reliability/soundness: Supervisory expectations from AI-driven models are similar to those for traditional models, but for AI models, assessing the reliability/soundness of model outcomes is viewed from the prospective potential of avoiding causing harm (e.g. discrimination) to consumers.
Some of the challenges in this context are related to technical issues like data quality, efforts for a regular and timely update when needed, existing regulatory requirements that are not fit-for-AI, and cyber risk issues.
2. Accountability: Similar to the traditional model requirement, but in the case of AI, human involvement is viewed more as a necessity. For AI models, accountability includes ‘external accountability’ to ascertain that data subjects (i.e. prospective or existing customers) are aware of AI-driven decisions and, if needed, that they have channels for recourse. The challenges posed in this area relate to lack of clarity around who is responsible at lower levels of hierarchy in the AI-driven models. Being alert to human induced risks, such as human introduced biases in the algorithm, is crucial when trying to avoid discrimination.
3. Transparency: This is perhaps the most important theme. The expectations here are also comparable to those for traditional models, i.e. explainability of decisions (the black box problem) and auditability of the decisions made. For AI models, external disclosure to data subjects is also expected (e.g. data used to make AI-driven decisions and how the data so used affects the decision).
The potential challenge is if a model is not transparent, supervisors cannot assess its reliability and hence cannot establish accountability. Also, supervisors often lack the technical skills to understand and explain such AI models.
4. Fairness: This means equitable treatment of consumers. It is crucial for AI models (although covered in existing regulatory standards, fairness expectations are not usually explicitly stated in traditional supervision standards). Expectations on fairness relate to addressing or preventing biases in AI models that could lead to discriminatory outcomes, but otherwise, ‘fairness’ is not typically defined.
5. Ethics: Stronger emphasis in AI models than in traditional models, where ethical expectations are covered but not explicitly applied (although covered in existing regulatory standards, ethics expectations are not typically applied explicitly to traditional models). Ethics expectations are broader than ‘fairness’ and relate to ascertaining that customers will not be exploited or harmed through bias, discrimination, or other causes. The challenges presented apply to ethics and fairness areas and relate to the lack of universally accepted definitions, regulations requiring human judgment, and financial exclusion (e.g. under-represented groups not receiving good credit scores as there is no past data). Also, human intervention may introduce human flaws and biases.
Drawing from these five themes, with the understanding that all the existing requirements on governance, risk management, and operation development for traditional models apply to AI models as well, there is a stronger emphasis on fairness, as avoiding biased/discriminatory outcomes requires human intervention. As the use of AI models that can impact authorities' conduct and prudential objectives increases, so too must increase the relevance of reliability, accountability, transparency, fairness, and ethics requirements for the supervision of these models.
EIOPA: Governance Principles for AI
EIOPA's report on governance principles on AI was developed by a stakeholder group on digital ethics and based on a survey conducted in 2018 on the use of AI in the European insurance sector. The survey showed that AI was mainly being used in sales and distribution, claims management, pricing. and underwriting. Efforts are underway for identifying use cases for AI across the insurance value chain. For an ethical and trustworthy framework to regulate AI driven models, the EIOPA report recommends the following principles:
1. Proportionality: The report provides an AI impact assessment framework to assess the impact of risk on consumers and firms, considering their severity and likelihood of harm.
2. Fairness and non-discrimination: This is the most extensively discussed principle in the report. Some key considerations that the report highlighted were:
- The need for taking into account the outcomes/decisions of AI systems
- Balancing different interests of the stakeholders involved
- Insurer's corporate social responsibility (allowing for financial inclusion issues and avoiding reinforcing existing inequalities)
- Respect for human autonomy
- Ensuring efforts to monitor and mitigate biases from data and AI systems by avoiding both direct and indirect discrimination
3. Transparency and explainability: The need for greater transparency and explainability in the use cases of AI.
4. Human oversight: Human supervision of the functioning of the AI model across the whole AI system's life cycle.
5. Data governance and record-keeping: The GDPR in Europe already includes a comprehensive framework for processing personal data.
6. Robustness and performance: This principle refers to the calibration, validation and reproducibility of AI systems soundly to ensure that AI systems' outcomes are stable, consistent and free of bias.
FSCA South Africa: DataEQ Case Study
Awelani Rahulani of the FSCA, South Africa shared the regulator's experience and insights gathered while using DataEQ, a ML driven tool for the analysis of social media posts, over the period of March-August of 2021. FSCA created a report on the performance and public perception of various insurance and other financial services solutions providers on the social web as analysed by DataEQ.
Through this solution, FSCA has tracked the public social media conversation around 214 Financial Service Providers (FSPs), observing that banking, long-term insurance products in particular and insurance, in general, contribute the largest volumes of conversations about FSPs on social media. The FSCA monitored consumer mentions containing themes related to their 'Treating Consumers Fairly framework,' measuring the completeness and transparency of financial advertising and customer treatment by FSPs.
The insights resulted in FSCA actions that would protect the public from further damage.
DataEQ has also been instrumental for FSCA's active communication, keeping the public informed about ongoing matters and driving public awareness on issues like unclaimed benefits.
By implementing these strategies, FSCA has been able to elevate the consumer's voice and hence protect the public against scams, in line with their vision of fostering a fair, efficient, and resilient financial system that supports inclusive and sustainable economic growth in South Africa.
Summary
In summary, AI-driven models and systems will transform the insurance industry. Insurance supervisors will have to evolve accordingly to supervise these models effectively and efficiently to protect consumers' interests. The work on identifying principles and thematic areas that will form the foundation of this evolution is well and truly underway. Supervisors need to keep track of them and actively adopt them. Perhaps most interestingly, AI will also offer supervisors the tools and mechanisms to help them in their tasks. This space will be exciting to watch as it evolves.
Share this article
Authors
Posts by Author
Topics Cloud
Subscribe to our list
Receive notifications when we publish new blog entries
Subscribe here